The field of medicine is undergoing a revolution, thanks to the potential of Artificial Intelligence (AI) in diagnostics and treatment. However, bias in AI has emerged as a significant concern, as it can lead to systematic errors in AI-generated outputs.
In this comprehensive article, we'll delve deeper into the causes of bias in AI for medical applications, its impact on healthcare, and the techniques you can use to identify, minimize, and manage these biases, all while making sure your head doesn’t spin.
So let’s get started…
What is bias in artificial intelligence?
Bias in AI refers to the presence of systematic errors in the outputs generated by AI algorithms, often resulting from training the algorithms on unrepresentative, skewed, or incomplete data. These biases can lead to unfair or inaccurate predictions and recommendations, potentially disadvantaging specific groups or individuals.
Why do we care about bias in AI, particularly in SaMDs?
Bias in AI can have significant consequences on the fairness, effectiveness, and overall trustworthiness of AI systems. In the context of medical applications, biased AI systems can lead to inadequate care or even harm to patients. Here are some reasons why we care about bias in AI:
- Fairness and Equity: Biased AI systems can exacerbate existing health disparities and social inequalities by providing inaccurate or biased predictions and recommendations for underrepresented groups
- Accuracy and Reliability: Bias can compromise the accuracy and reliability of AI-generated outputs. In medical applications, this can result in misdiagnoses, incorrect treatment recommendations, or other adverse outcomes for patients.
- Ethical Considerations: The use of biased AI systems raises ethical concerns, as it can perpetuate discrimination and unfair treatment of specific groups or individuals.
- Legal and Regulatory Compliance: Ensuring that AI systems are unbiased and fair can help organizations comply with laws and regulations that prohibit discrimination based on factors such as age, gender, ethnicity, or disability.
- Public Trust and Adoption: Addressing bias in AI systems builds public trust and promotes the widespread adoption of AI technologies. If people perceive AI systems as biased or unfair, they may be less likely to use or trust these technologies, hindering their potential benefits across various industries, including healthcare.
- Economic Impact: Biased AI systems can lead to suboptimal decision-making and resource allocation, affecting organizations' bottom lines.
So, what are the main types of bias in AI?
Bias in AI can be categorized into three main types: unrepresentative data, skewed data, and incomplete data.
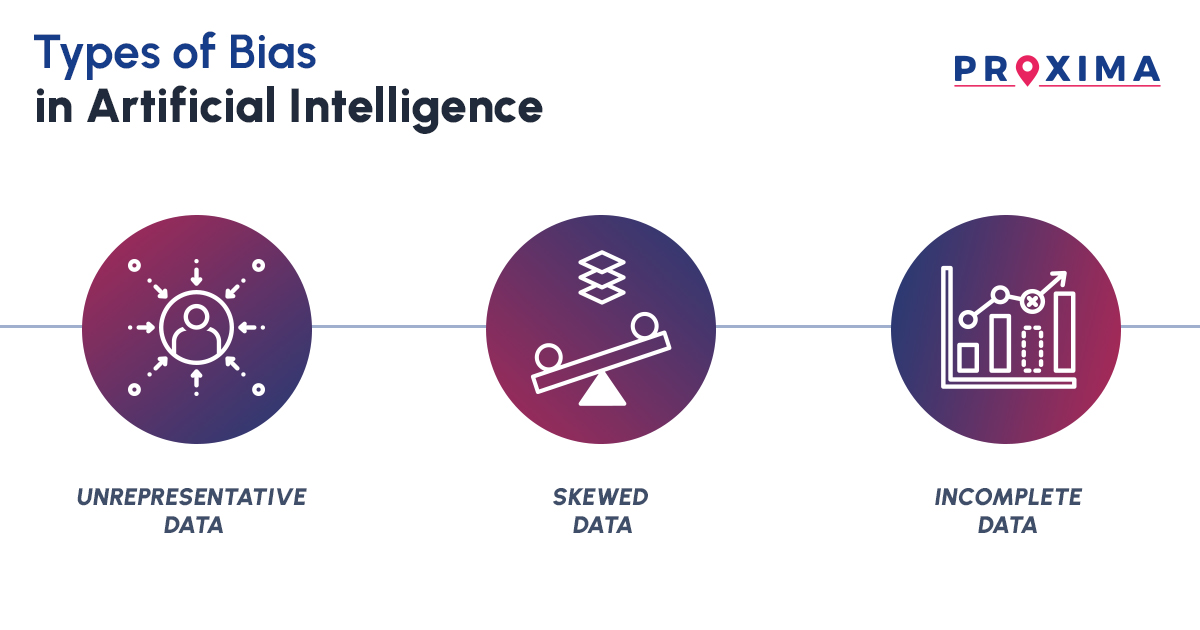
- Unrepresentative Data: Unrepresentative data refers to when the training data doesn’t adequately represent the intended patient population, their medical conditions, or other relevant factors that the AI system would encounter in the real world. This can result from issues such as limited sample size, biased sampling, skewed data, or temporal and geographical differences.
- Skewed Data: Skewed data occurs when certain groups or conditions are over- or under-represented in the training data, leading to biased predictions. This imbalance can cause the AI system to generate predictions that may favor over-represented groups and perform poorly for under-represented groups.
- Incomplete Data: Incomplete data refers to a dataset where important information is missing, such as specific variables, data points, or records. This missing information could be due to data collection errors, data entry mistakes, or the unavailability of certain data elements. Incomplete data can hinder an AI system's ability to make accurate predictions or recommendations.
What causes bias in AI?
Bias in AI arises from three main causes: data imbalance, data quality issues, and algorithmic limitations.
- Data Imbalance: Data imbalance occurs when the training data for AI algorithms is unrepresentative, skewed, or incomplete, as mentioned above. This can happen when certain patient groups or conditions are over- or under-represented, leading to biased predictions and exacerbating existing health disparities. Examples include demographic imbalance, underrepresentation of rare diseases or specific medical conditions, and biased selection of hospitals or locations.
- Data Quality Issues: These issues arise when training data is inaccurate, outdated, or inconsistent, affecting the AI system's reliability. Examples include data entry mistakes, inconsistencies in data collection methodologies, outdated medical knowledge or guidelines, noisy data, and unreliable or low-quality sources.
- Algorithmic Limitations: Algorithmic limitations refer to issues with the AI model itself, such as inappropriate feature selection, model design, or biased assumptions. Balancing the model's complexity between highly complex and overly simplistic designs is a significant challenge.
Techniques to Detect and Address Bias:
Various techniques, such as statistical methods, data visualization, model interpretability techniques, and the involvement of diverse teams, can be employed to effectively identify and quantify biases in AI systems. As a developer, you can then address potential shortcomings and improve the overall performance, fairness, and accuracy of AI algorithms across different applications. Here are some more details on each of these.
- Statistical Methods: Statistical methods, such as hypothesis testing or measuring disparities in performance metrics across different groups, can help determine if there are significant differences in AI system predictions for various subpopulations. Comparing performance metrics across different groups can reveal disparities that might indicate bias.
- Data Visualization: Visualizing data can reveal imbalances or discrepancies. Heatmaps, bar charts, and distribution plots can be used to assess the AI system's performance across different patient demographics or medical conditions. Distribution plots can help visually assess whether the training data is representative of the target population.
- Model Interpretability Techniques: Feature importance analysis, partial dependence plots, or SHAP values can help explain the AI model's decision-making process and uncover potential biases in its predictions. Techniques like permutation importance and SHAP values provide insights into the contribution of each feature toward the prediction, enabling a deeper understanding of the AI model's behavior.
- Diverse Teams: A diverse team of engineers, scientists, clinicians, and regulatory experts can help identify and address potential sources of bias in AI systems for medical devices. The diversity of backgrounds, expertise, and experiences leads to unique ideas and insights, fostering collaborative problem-solving and minimizing blind spots.
Strategies for Reducing Bias:
Let’s say you’re a developer looking to reduce bias in AI for medical applications. Well, you can employ various data collection, curation, and algorithmic improvement strategies, such as:
- Gathering Data from a Broad Range of Sources: You should collect data from various sources, such as various electronic health records, medical imaging data from different systems, and patient-generated data. This ensures a more comprehensive and representative dataset, reducing the likelihood of bias.
- Balancing the Data: You can use oversampling or undersampling techniques to balance the data and ensure it is representative of all patient groups and conditions. Oversampling increases the number of samples for the minority group while undersampling reduces the number of majority class samples to achieve a more balanced dataset.
- Cleaning and Preprocessing the Data: Cleaning and preprocessing the data ensure high-quality, error-free datasets. This process involves removing duplicate records, filling in missing values, and normalizing the data, similar to data management in clinical trials.
- Algorithmic Improvements: You can apply techniques like regularization, fairness constraints, and other algorithm-specific improvements to enhance accuracy, and fairness, and reduce bias. The choice of techniques will depend on the type of algorithm and the specific issue being addressed.
- Ongoing Testing and Evaluation: Continuous monitoring and evaluation help to maintain the accuracy, reliability, and effectiveness of AI medical devices. Establish clear evaluation criteria, such as accuracy, sensitivity, specificity, and positive predictive value. These criteria should be based on the device's intended use case and patient population.
You should also monitor the AI medical device's performance over time through automated monitoring systems or regular quality control checks. This ongoing assessment helps identify potential biases and performance issues that may arise, ensuring that your AI systems continue to provide reliable, unbiased outputs in medical applications.
The Bottom Line:
Bias in AI for medical applications is a complex issue that requires a multifaceted approach to address effectively. If you’re a developer, understanding the root causes of bias, employing techniques to detect and minimize it, and continuously evaluating AI system performance, you can create AI medical devices that provide accurate, reliable, and unbiased predictions and recommendations.
By, aligning yourself with an experienced and trustworthy CRO like Proxima, you can leverage expertise in data collection, trial planning, and analysis to effectively carry out these techniques and mitigate the consequences of biases in your AI systems. With our robust quality assurance processes, adherence to ethical and regulatory guidelines, and commitment to transparency, you can continue to harness the power of AI while ensuring fair and equitable outcomes for all patients.